Integrating Big Data with Graph Theory to Model Complex Transportation Systems
Research and Analysis Journal
Vol. 5 No. 6 (2022),Volume
2022
,
Page 01-27
https://doi.org/10.18535/raj.v5i6.294
Abstract
The rapid growth of urbanization and globalization has amplified the complexity of modern transportation systems, necessitating innovative approaches to model and optimize these networks. This study explores the integration of Big Data and graph theory as a robust framework for analyzing and managing complex transportation systems. By leveraging vast datasets sourced from IoT devices, GPS trackers, and social media, the research harnesses the power of graph-based models to represent transportation networks with high fidelity.
Key methodologies include the application of dynamic graph algorithms to capture real-time traffic patterns, congestion hotspots, and route optimization. Additionally, machine learning techniques are integrated to enhance predictive capabilities and scalability. The study highlights practical applications in urban transit planning, logistics management, and smart city development.
Challenges such as data heterogeneity, computational scalability, and privacy concerns are also addressed, with proposed solutions for future advancements. The findings underscore the transformative potential of combining Big Data with graph theory, paving the way for more efficient, adaptive, and sustainable transportation systems in an increasingly interconnected world.
- Big Data, Graph Theory, Transportation Systems, Network Modeling, Real-Time Analysis, Traffic Optimization, Dynamic Graphs, Machine Learning, Smart Cities, Predictive Analytics, Urban Transit Planning, IoT in Transportation.
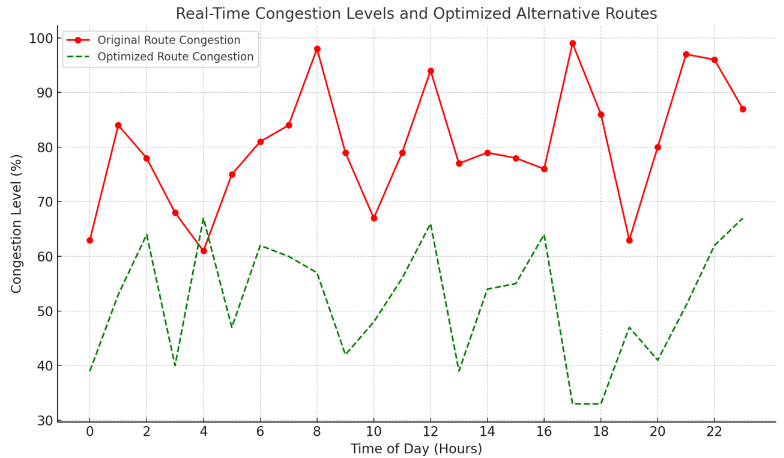
How to Cite
Download Citation
References
- Article Viewed: 17 Total Download